Get the White Paper
Client-side AI: Privacy, performance, and cost advantages in modern browsers
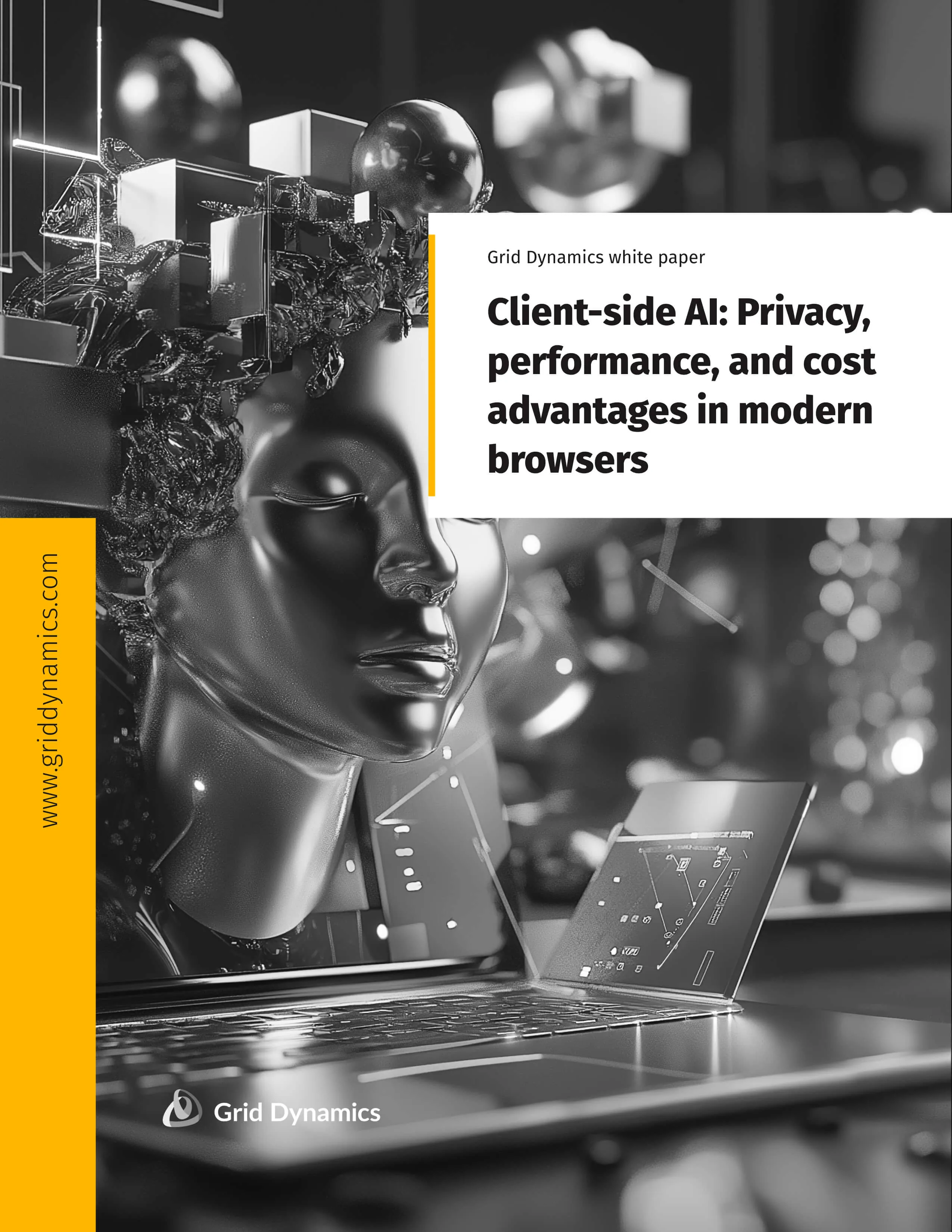
The future of fast, secure, and scalable web apps
Download the white paper to get your hands on a comprehensive guide on the privacy and performance benefits, as well as implementation, optimization, and security best practices of client-side AI. Below is a taste of what you can expect, with more in-depth details, code samples, and actionable strategies available in the full white paper.
What is client-side AI?
Artificial Intelligence (AI) has traditionally relied heavily on server-side processing, requiring significant computational power and substantial infrastructure investments. However, with recent advancements in browser technologies, such as WebGL, WebAssembly, and WebGPU, it has become increasingly practical to run complex AI models directly within the user’s browser—often called client-side AI. This approach, often termed client-side AI, transforms browsers into powerful computing environments capable of efficiently executing machine learning (ML) and neural network models.
Deploying AI in browsers addresses critical challenges related to latency, privacy, scalability, and offline accessibility. Users gain instant, responsive interactions without delays from network requests, ensuring a smoother and more personalized experience. Furthermore, by keeping data processing local, this method enhances privacy and security, significantly reduces server costs, and offers scalable performance as each user’s device contributes computing resources. The shift toward browser-based AI reflects a broader industry trend aimed at delivering responsive, private, and efficient user experiences directly on client devices.
Benefits of client-side AI
Running AI directly in a browser or client-side AI is becoming increasingly relevant, thanks to several key technical and practical benefits:
Privacy-preserving AI and security
- User data never leaves the browser, ensuring sensitive data stays local.
- No need to send potentially confidential data to external servers, reducing security risks.
📒Example: A user uploads an image for face recognition, and all processing occurs on their device.
Real-time performance
- Eliminates delays (latency) caused by network requests.
- Enables smooth, real-time interactions, essential for applications like gesture recognition, face tracking, or Augmented Reality (AR).
📒Example: Gesture-based interactions in a web app responding immediately without waiting for server processing.
Reduced server side costs and load
- Offloading computational tasks to user devices minimizes server workloads and reduces infrastructure costs.
- Scalability improves dramatically as users bring their computational resources (CPU/GPU).
📒Example: Processing thousands of simultaneous users for image filtering or classification without additional server expenses.
Offline capabilities
- Enables AI-powered applications to function fully offline.
- Users can rely on consistent performance even without an internet connection.
📒Example: A browser-based image editor using AI to enhance photos offline.
How browser-based AI works: From model loading to client-side inference
From a technical perspective, running AI in the browser implies:
- Client-side execution of AI models: Neural networks or other ML models are loaded directly into the browser memory and executed locally, usually using JavaScript or browser-native APIs.
- Direct use of browser capabilities: Utilizes browser APIs, such as WebGL, WebAssembly, and WebGPU, to execute computationally intensive tasks, harnessing the device’s GPU/CPU directly.
- Model optimization and deployment: AI models are optimized for size and speed (via quantization, pruning, and compression techniques) to ensure smooth performance within browser resource constraints.
- Library and frameworks for AI in browsers: Frameworks such as TensorFlow.js, ONNX.js, or MediaPipe provide ready-made solutions for deploying trained ML models easily and efficiently.
📒Technical example: Loading a pre-trained image classification model (TensorFlow.js) into browser memory, executing predictions using WebGL acceleration, thus providing instant, client-side image classification without a server round-trip.
Leveraging modern web technologies and frameworks like TensorFlow.js, ONNX.js, and MediaPipe for client-side AI
As web applications become increasingly sophisticated, there’s a growing demand for executing complex AI tasks directly within browsers. Recent advancements have significantly expanded the browser’s computational capabilities, enabling technologies like WebGL, WebAssembly, and WebGPU to efficiently run machine learning models locally. Understanding these technologies and their browser compatibility is crucial for leveraging client-side AI effectively.
Running AI efficiently inside the browser demands specialized technologies that leverage local computational resources (CPU/GPU). Three key players are WebGL, WebAssembly, and WebGPU:
Technology | Description | How it helps with AI | Common use-cases | Frameworks |
---|---|---|---|---|
WebGL | A JavaScript API that gives direct access to a device’s GPU primarily for rendering 2D and 3D graphics—but also highly effective for accelerating AI computations. | WebGL can significantly speed up neural network computations by parallelizing tensor operations directly on the GPU. | – Real-time image/video processing.– Object detection, face detection, and pose estimation. | – TensorFlow.js: Utilizes WebGL for accelerated neural network inference.- Brain.js, ConvNetJS: Lightweight neural network libraries leveraging WebGL for fast execution. |
WebAssembly (WASM) | A binary instruction format allowing browsers to run code written in languages like C, C++, or Rust, providing near-native performance directly in-browser. | WebAssembly enables computationally intensive AI operations (such as complex math or heavy models) to execute quickly and efficiently, significantly outperforming pure JavaScript implementations. | – Complex model inference (e.g., large image classifiers).– Advanced numerical operations and simulations. | – ONNX Runtime Web: Runs optimized ONNX models with high performance in browsers via WASM.- MediaPipe: Google’s AI-powered solution using WASM for real-time computer vision tasks (face/hand tracking). |
WebGPU | A modern web standard for high-performance GPU computations, designed explicitly for GPU-intensive tasks like AI. WebGPU is positioned as a successor to WebGL, providing improved performance, lower overhead, and better GPU resource control. | WebGPU dramatically enhances GPU-based calculations. It provides optimized execution of AI models, enabling more complex models to run faster and more efficiently than WebGL. | – Highly intensive ML tasks (e.g., GANs, Stable Diffusion models).– Real-time ML-powered rendering and AR/VR applications. | – TensorFlow.js (WebGPU backend): Significantly boosts ML inference performance. – Emerging experimental tools and libraries optimized specifically for WebGPU. Transformers.js v3 pyGandalf,WebLLM |
Comparison of WebGL, WebAssembly, and WebGPU web technologies
To effectively evaluate and compare WebGL, WebAssembly, and WebGPU, consider the following key metrics relevant to running AI models in browsers:
Metric | WebGL | WebAssembly | WebGPU |
---|---|---|---|
Performance(how fast and efficiently it runs AI computations) | ✅ Good GPU acceleration, moderate efficiency. Ideal for standard ML tasks. | ✅ Near-native CPU performance. Excellent for computationally intensive numerical tasks. | ✅ Highest GPU performance. Optimal for complex, heavy AI models requiring intense parallel computations. |
Ease of development(simplicity and clarity of implementing AI solutions) | ✅ Moderate complexity; mature frameworks available (e.g., TensorFlow.js). | ✅ Higher complexity; involves compiling from languages like C++/Rust, demanding deeper engineering expertise. | ⚠️ Currently complex due to emerging nature and limited documentation, but expected to improve. |
Browser support(compatibility across different browsers) | ✅ Excellent, universally supported across modern browsers. | ✅ Excellent, widely supported across modern browsers. | ⚠️ WebGPU support is limited but rapidly growing; supported by latest versions of Chrome, Edge, and Safari (experimental support). |
Stability/Maturity(reliability and readiness for production) | ✅ Mature, stable, and production-ready. | ✅ Mature, stable, well-supported in production environments. | ⚠️ Early-stage, experimental; recommended for prototyping and exploring future-ready projects. |
Summary
- Use WebGL when immediate compatibility, ease of web development, and stable GPU acceleration matter most. It’s the most practical choice for many existing AI tasks in browsers (e.g., real-time classification, image processing).
- Choose WebAssembly when you need maximum CPU performance, have complex numerical models, or when your AI models can’t effectively leverage GPUs. Especially beneficial if you already have code samples in C/C++/Rust.
- Consider WebGPU as your future-oriented choice. Ideal for forward-looking projects requiring intensive GPU computations (like generative AI, agentic AI, natural language processing, large language models, advanced visualizations, or real-time ML rendering). Keep an eye on its development and start experimenting early.
Cross-browser compatibility assessment
Why is cross-browser compatibility important?
Different browsers handle web technologies (like WebGL, WebAssembly, WebGPU) differently. Compatibility ensures all users, regardless of their browser choice, have consistent access to AI features.
Compatibility overview
Technology | Chrome | Firefox | Safari | Edge | Notes |
---|---|---|---|---|---|
WebGL | ✅ Full | ✅ Full | ✅ Full | ✅ Full | Widely supported, safe choice |
WebAssembly | ✅ Full | ✅ Full | ✅ Full | ✅ Full | Excellent support, reliable |
WebGPU | ✅ Partial (experimental) | ⚠️ Limited (behind flag) | ✅ Partial (experimental) | ✅ Partial (experimental) | Emerging standard, careful testing needed |
Conclusion
Running AI directly in the browser (client-side AI) brings significant benefits, including increased user privacy and data privacy, reduced latency, cost-effective scalability, and offline capabilities. Key technologies like WebGL, WebAssembly, and WebGPU play crucial roles, each suited to specific performance and compatibility requirements. To maximize AI performance within browsers, developers should use optimization techniques such as quantization, pruning, and efficient architectures.
However, browser-based AI introduces specific constraints like model size, memory limitations, and cross-browser compatibility challenges. Addressing these effectively involves leveraging proven libraries, practical boilerplates, adaptive design principles, and robust testing strategies, including AI-driven approaches.
Security and privacy remain paramount, requiring diligent practices such as HTTPS delivery, content security policies (CSP), data minimization, regular vulnerability assessments, and explicit user consent. By adhering to these recommendations, developers can confidently build performant, secure, and user-friendly AI-powered web experiences.
Tags
You might also like
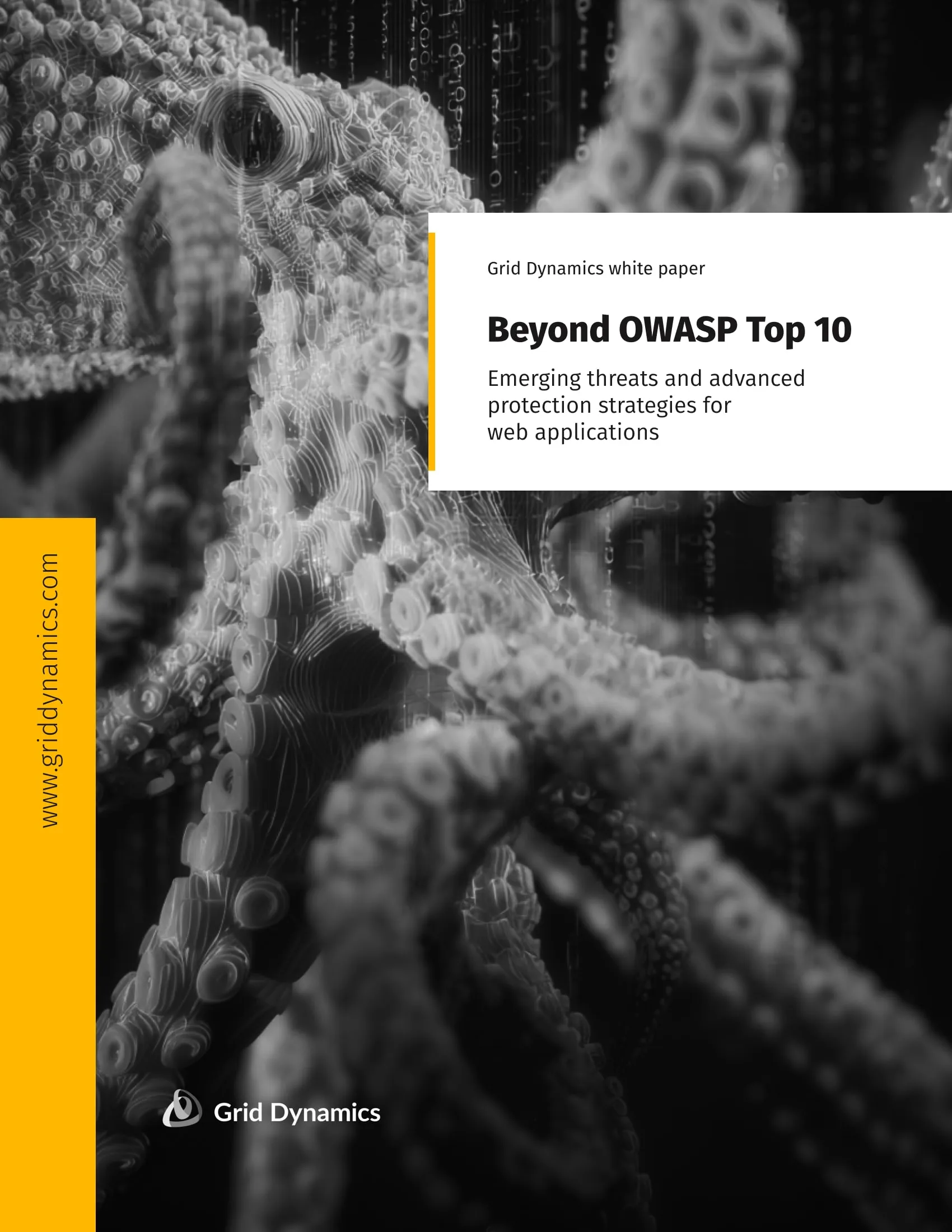
Download this white paper for comprehensive details on how large-scale applications can overcome web application security risks and evolving web threats, including AI-driven attacks, supply chain vulnerabilities, and compliance pitfalls. It goes beyond traditional checklists for web applicati...
From performance and portability to real-world limitations, this white paper explores how WebAssembly (Wasm) is modernizing web development and its growing role in handling AI. Download the full WebAssembly white paper if you’re a frontend and full-stack developer, CTO or technical lead, D...
From retail to manufacturing, and from financial services to healthcare, every industry is eager to capitalize on the potential of artificial intelligence. But AI-ready data is essential to realizing that promise. To truly unlock that potential, AI solutions for enterprises must be built on a fou...
Delivering reliable software at speed is challenging. Even more challenging is continuing to rely on traditional quality assurance as digital transformation accelerates. Manual testing and conventional test automation simply can't keep up with the complexity and pace of modern development. Arti...
Is DeepSeek AI development the right choice for your organization? Download the full white paper to get your hands on comprehensive technical details, in-depth performance benchmarks, and actionable insights from CTOs—for CTOs (and AI innovators). DeepSeek has quickly established itsel...
This white paper explores how Vercel frontend deployment innovations, including developer experience optimization, fluid computing, and AI-assisted development, help you accelerate development velocity by 30-50%, improve global performance by 30-50%, and reduce infrastructure management overhea...
For technical leaders seeking a comprehensive understanding of Agentic AI technology—including architectural innovations, implementation frameworks, and detailed technical guidance—download the full white paper for an in-depth analysis, technical deep dive, and actionable strategies to accelera...