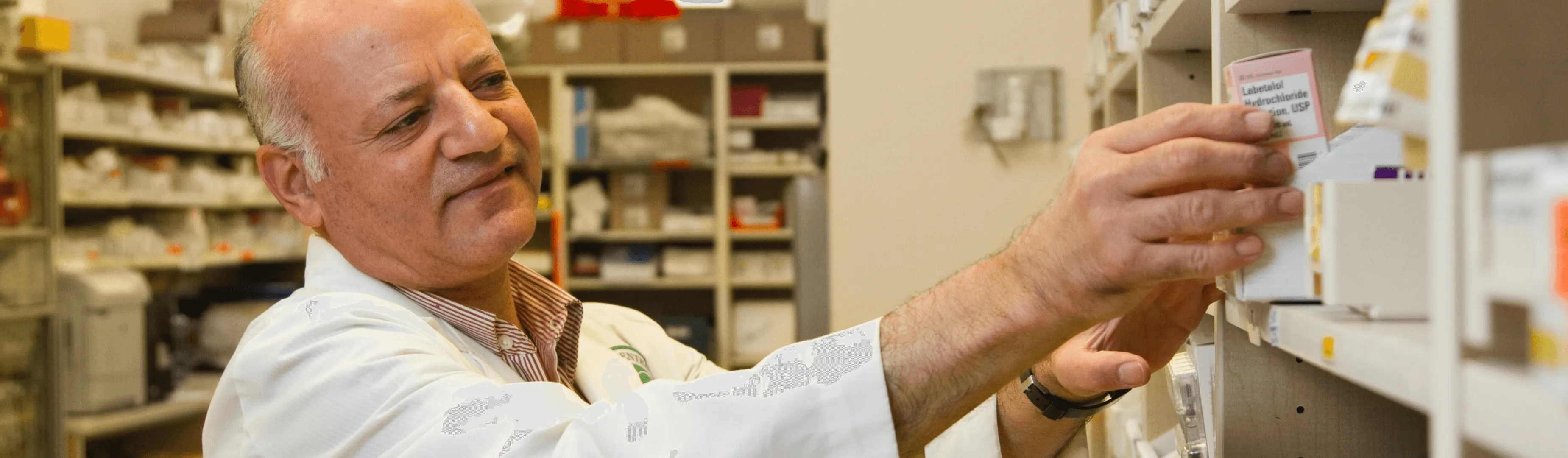
Operationalizing next best action in commercial pharma: A blueprint
August 2, 2022 • 9 min read
- Harmonized communication for impactful marketing
- Mapping NBA solution capability to business outcomes
- The model: NBA for channel likelihood and channel values
- Business workflow of an NBA model in a commercial pharma setting
- NBA blueprint
- NBA reference architecture and tech stack
- Business value for a commercial pharma client
- Lessons learned
There is no doubt that customer-centricity and personalization tools are driving digital transformation investments in commercial pharma. The big question, however, is how to operationalize data-driven insights that help pharma sales reps overcome the challenge of limited access to health care providers (HCPs).
The answer lies in machine learning enabled solutions that aid in the creation of an integrated promotion plan in which field forces, reps and marketing teams collaborate to reach HCPs via the right channel, at the right time, and with the correct content, thereby increasing the promotional impact. This article delves into the blueprint of NBA and its functions in a commercial pharmaceutical setting.
Harmonized communication for impactful marketing
Historically, field forces and marketing teams have functioned independently. Sales reps contact HCPs to inform them about a product, yet the same HCP may receive a corporate email from headquarters on a different product, resulting in disconnected messaging. These random emails bombard HCPs with an excessive number of touchpoints each day, resulting in what can be seen as a disorganized and impersonal communication process – a prevalent trend for commercial pharmaceuticals.
These communication challenges can be overcome by developing comprehensive marketing strategies and employing advanced ML techniques. Next best Action (NBA) can be created to recommend individual HCP-level content and channels based on all past interactions and touchpoints. The NBA engine converts past interaction data and campaign settings into recommendations, such as recommending the optimal channel for the next HCP engagement. Additionally, it may propose the optimal time to send corporate emails for optimal interaction and notify the field teams using automated marketing platforms. Consequently, marketing teams may move their focus to curating smart content, lowering the risk of time and money spent on marketing while increasing consumer satisfaction and revenue. NBA is effective because it can customize channels and content based on HCP affinity, as well as synchronize and arrange the order and weight of suggestions. NBA is highly adaptive and can modify its suggestions based on the customer’s every recent action; it can not only suggest the next best action to reps, but also offer the rationale for suggested channels and material.
Mapping NBA solution capability to business outcomes
NBA engines represent a new paradigm for building customer analytics and personalization systems that take a customer-centric approach to optimization of HCP engagement, using innovative modeling techniques to overcome the limitations of traditional personalization methods. The solution capabilities of NBA include analysis of HCP behavioral drivers, optimization of action sequences and interaction parameters, and development of interactive learning based on real-time events. These capabilities result in higher engagement, shorter time-to-engage, reduced negative outcomes (unsubscriptions or over saturations), and increased value delivery to HCPs.
The model: NBA for channel likelihood and channel values
NBA can be used for omnichannel pharma marketing strategies that involve using a variety of communication channels to promote numerous products/drugs to HCPs. As a result, given an objective function, the NBA model can rank various channels and products based on HCP data.
The objective function is represented as the expected impact of each action on the final objective, and the final objective can be any acceptable marketing indicator (KPI) for measuring success.
For instance, an HCP opening an email sent by a rep (action) is projected to increase HCP engagement (end goal) by 0.1, whereas an HCP accepting a face-to-face meeting invite (action) from a rep boosts engagement (goal) by 0.2. Therefore, for this campaign, face-to-face meetings seem to rank higher. We hypothesize that we may improve HCP engagement (goals) by recommending channels (actions) that HCPs are more likely to engage with (objective function). These weights are hypothesized either heuristically or based on the importance of the channel. Marketing teams can get this information from their business intelligence dashboards or past channel performance experience.
- Related article: KPIs for pharmaceutical manufacturing operations
Objective function = HCP engagement
Actions = HCP open email rate, HCP acceptance of calls and face-to-face meeting, HCP acceptance to webinar invites, HCP’s deep link click rates etc.
Goal = Maximize (HCP engagement)
A wide array of channels are used for promotional efforts in the commercial pharma industry. The channels are chosen with the purpose of increasing HCP engagement. The difficulty with such an omnichannel approach is how to connect with HCPs through all relevant channels without bombarding them with too much communication. With an NBA model, sales reps are able to prioritize and potentially restrict promotions based on HCP interaction history, preferences, and specializations, making the communication more value-driven than volume-driven. Before getting into the deeper workings of the model, to gain more perspective, let us look at the business set-up, channel content, and workflow that NBA can implement.
Business workflow of an NBA model in a commercial pharma setting
There are a variety of interactions that occur between sales reps, pharma headquarters and HCPs, as well as a variety of channels for these interactions. Common channels usually include:
- Corporate emails – these emails are sent by the pharma company headquarters as part of marketing initiatives. These are often campaign-driven and do not involve a sales rep. These communications are not customized at the individual level and generally target a broader segment of HCPs.
- Sales rep emails – These emails are sent to HCPs by sales reps and field forces. These are more tailored emails that have been meticulously prepared by reps based on their relationship with HCPs.
- Invitation to a webinar – Sales reps often invite HCPs to specific events and webinars. These campaigns are semi-segmented depending on the demographic information of HCPs, such as specialties, location, etc.
- Face-to-face meetings are pre-arranged meetings in which reps visit with HCPs in person to discuss products or distribute pertinent information.
- Calls – Sales reps often schedule phone appointments with HCPs to discuss products and create connections.
When a sales rep is ready to communicate with an HCP they usually choose one or more channels to do so. If the rep chooses a channel that the HCP is unlikely to engage with, the effort put into communicating is wasted. TO solve this challenge, our NBA engine recommends the channels that HCPs are most likely to engage with.. These suggestions are formulated based on input data from Veeva and Salesforce, which contain HCP demographic information, interaction history, and consent to contact data. The NBA model employs business criteria, optimization function and channel ranking to determine the optimal channel for interaction. Business rules are required for orchestrating NBA because they define campaign specifics such as HCP segmentation, the number of emails an HCP can receive within a given time period, etc. Additionally, NBA includes justifications for each suggestion for added clarity. Sales reps then have the option to either accept the suggestion and proceed with the content and channel recommendations, or they can reject the suggestion and provide feedback as to why it was rejected. This feedback is used to train the model for future suggestions.
The diagram below shows the business workflow that NBA uses to suggest channels.
Historical data used in the NBA model
HCP data, rep data and content links are the primary type of data necessary for our NBA engine. A sample of input data and key features are tabulated below.
A big part of the NBA model involves:
- Extensive research on channels;
- Marketing goal alignments;
- Campaign constraints;
- HCP info;
- HCP journey touchpoints;
- The rep’s objective.
Investigating the correlation between channels and HCP engagement is a crucial activity. HCP consent to contact data acts as a primary filter on NBA. We identify HCP behavioral affinity to specific channels more frequently than others. The end goal of NBA is to optimize HCP engagement, with an objective function that consists of two components – the channel value and the channel likelihood. The objective function needs to represent each channel’s expected impact on HCP engagement.
Channel value is a measure of the expected channel impact on HCP engagement. Channel value should answer the question: “How will HCP engagement change if I start engagement with channel A?”
Channel values are calculated on an individual HCP level, so there is a single value per channel on a given instance. The channel value for each campaign is periodically re-calculated and updated to ensure that recommendations adapt to potential changes in HCP channel preference.
Channel likelihood is the probability that an HCP will engage with a given channel. This is periodically calculated separately for each HCP and is ranked with a value from 0 to 1. This is achieved by training a model for each channel, taking into account features from the past 12 months, and predicting interaction for the following campaign duration. Features used to make the prediction include an analysis of HCP interaction with other channels available and recent HCP engagement
Pre-defined weights are then applied to both components, which are then combined to get a ranking of all channels for each HCP. This is then loaded to some type of database file system and displayed on a dashboard or some type of UI where sales reps can access the data in the form of recommendations.
NBA blueprint
The NBA core consists of three layers: data processing, AI/ML, and orchestration. For implementation, we add two other levels to the design architecture – landing zone, and integration & feedback layer, taking into account the numerous components necessary to fulfill the suggestion work in production environments.
Starting with the core NBA engine and its inner workings, the diagram above displays the NBA engine’s central part.
1. The data processing layer preprocesses a vast quantity of various datasets retrieved from marketing cloud platforms, Veeva, Web Analytics data, and other event-related data such as webinars or other event information. The Veeva and Salesforce marketing systems include past Rep-HCP interactions, HCP profile information, HCP data, HCP touchpoints, channel data, event and webinar data, and contact data. These data fields are feature-engineered using principal component analysis (PCA) techniques to choose the most important feature set for modeling.
2. The AI/ML layer comprises HCP behavior driver analysis, propensity scoring, and strategic decision making. Models of behavior drivers include models for the study of event sequences, such as sales rep-sent emails, approved emails, and invites to events and phone conversations. These models enable the development of enhanced reports that objectively identify the drivers of HCP interaction with rep and HQ operations based on historical engagement patterns. Propensity models provide a collection of tools for rapidly developing individualized propensity models that quantify how affinity to channels, indicators, or content evolves over time.
3. The orchestration layer handles the decision engine that combines the scores provided by the propensity models, customer value considerations, and strategic (multi-step) optimization to determine the optimal best action. The engine can function interactively, autonomously making decisions and learning from reps’ real-time feedback. This engine also considers the business rules and campaign limits specified by marketing teams.
4. The landing zone layer is used to create a secure, compliant, multi-account AWS environment. Construction of a landing zone offers numerous advantages, such as:
- reduced manual effort;
- improved quality, and faster service delivery;
- enhanced scalability, security, and governance;
- increased network resilience and performance;
- decreased operational cloud costs;
- increased operational rigor through a shared service model; and
- increased agility through a well-defined operating model.
AWS Config rules are enabled to monitor Amazon Elastic Block Store, Amazon S3, Amazon Relational Database Service storage encryption, AWS Identity and Access Management (IAM) password policy, root account multi-factor authentication (MFA), Amazon S3 public read and write, and insecure security group rules. In addition, HCP information, rep information, and other data sources are accessible through the landing zone from S3 buckets containing HCP information and rep information.
5. The integration and feedback loop layer is where recommendations for the field force are applied. It can integrate into the Veeva platform, a marketing cloud platform, or a user interface that reps have access to. The feedback layer gathers sales rep comments about the NBA channel recommendations; for example, if a rep feels that an unsuitable channel was recommended, they have the option to provide feedback on why the channel is unsuitable. Rep suggestions are then used to improve the NBA model for future learning purposes.
NBA reference architecture and tech stack
This is an example of NBA architecture that is built on AWS. The NBA engine can also be implemented in other cloud platforms or deployed as a stand-alone solution.
Business value for a commercial pharma client
We implemented an NBA solution for a commercial pharma client who was experiencing HCP marketing challenges. To continuously augment the solution, every piece of content is labeled after the marketing team identifies the most critical campaigns and establishes communication priorities. This data, along with all previous HCP interaction data, is then loaded into the NBA engagement tool, which provides daily suggestions to sales reps about the next best channel to employ. Once the sales rep implements a recommendation, the marketing and sales teams can measure the impact of the action and adjust their approach appropriately, continually becoming more intelligent and in tune with their consumers.
In addition to expected performance-improvement, the organization became more responsive to market shifts. The system is updated with new data every day, which enables sales reps and the company as a whole to pivot swiftly. The NBA solution is also very scalable and adaptable and can be used across industries and regions.
Lessons learned
It is critical to conduct the NBA process step by step; attempting to do it all at once would undoubtedly fail. Begin by tagging all pieces of content, links, and emails to ensure that they can be completely tracked, and then gather the data and develop the model from there.
Another crucial step is to start simple. With one or two promo products and a handful of channels, develop a POC and monitor the model for a specific trial period. Then, including a high-level executive, having a key sponsor will assist you in expanding the NBA algorithm to other areas.
In the next blog, Designing an objective function for personalization in pharmaceutical marketing, we discuss yet another NBA model that uses the customer engagement index (CEI) to rank channels for recommendations.
Feel free to get in touch with us to start a conversation about operationalizing NBA in your organization.
LEARN MORE
Generative AI in pharma