5 PB
covered data
1, 000s
covered datasets
10x
reduction of data defects
Real-time
monitoring
AI-powered
anomaly detection
PRODUCTION DATA QUALITY MANAGEMENT
Increase confidence in data and insights
As companies become more data-driven, the cost of errors due to bad data increases. Corrupted data leads to poor quality reports and disastrous AI decisions, eroding business stakeholders’ confidence in data and the accuracy of insights. Adding data quality monitoring and management to the production data lake helps detect, prevent, and auto-correct data defects and ultimately leads to data quality assurance. Data quality assurance means reaching more relevant insights, better decision making, boosted business values, and increased trust from stakeholders.
BUSINESS RULES ENFORCEMENT
Detect data corruption and prevent it from spreading
Automatic business rules validation is the most reliable way of achieving good data quality. The best data quality tools will integrate with any data engineering technology stack by injecting business rules enforcement jobs between critical data processing and transformation jobs. A convenient graphical interface will decrease implementation costs by reducing the amount of coding the team has to do and empowering your data analysts.
ANOMALY DETECTION
Rely on AI to find unusual patterns
It’s not always possible to define business rules to enforce data quality at every step of the data processing pipeline. AI-powered anomaly detection automates data quality and helps scale it to thousands of data pipelines with minimal effort. From basic statistical process control to deep learning algorithms, AI learns the relevant data profiles in real-time, uncovering hidden defects or unusual patterns. A rich user interface helps tune and monitor data quality metrics and profiles, allowing data scientists to achieve a deeper understanding of the data.
DATA CONSISTENCY CHECKS
Ensure consistency with systems or record
Poor data quality is often caused by issues with data ingestion. Common issues include missing, corrupted, or stale data. Stream ingestion and processing can increase the chances of sourcing inconsistent data due to missed events. Adding consistency and completeness checks between raw datasets and systems of record improves data quality early in the pipeline, preventing corruption from entering into the system.
AUTOCORRECTION AND SELF-HEALING
Autocorrect data defects
In some cases, it’s possible to achieve automatic correction of data issues. Similar to the business rules that detect data corruption, injecting auto-correcting rules helps self-heal and avoids downtime.
Data Observability Starter Kit
The Data Observability Starter Kit simplifies data quality onboarding for modern businesses by offering checks for tabular, structured, and unstructured data. It includes built-in quality assessments for null/missing values, statistical distributions, data freshness, volume, and anomaly detection through unsupervised learning models. Easily integratable into data platforms and modern data warehouses, this starter kit ensures a swift time-to-market for monitoring data quality across all data types.
Our clients
RETAIL
HI-TECH
MANUFACTURING & CPG
FINANCE & INSURANCE
HEALTHCARE
Get to market faster with our data quality starter kit
We have helped Fortune-1000 companies improve their data quality in the most demanding data platforms. This includes platforms holding 5+ petabytes of data, processing hundreds of thousands of events per second, across thousands of datasets and data processing jobs. This provided us with the expertise to develop a complete set of data quality management tools as part of the development of our starter kit. The starter kit is based on an open-source cloud-native technology stack and is infrastructure agnostic – with the ability to deploy in AWS, Google Cloud, or Microsoft Azure. It integrates best with Hadoop and Spark-based data lakes with Apache Airflow orchestration, but also supports integration with SQL-based data sources out of the box and integrates with any other analytical data platforms, data warehouses, databases, and ETLs.
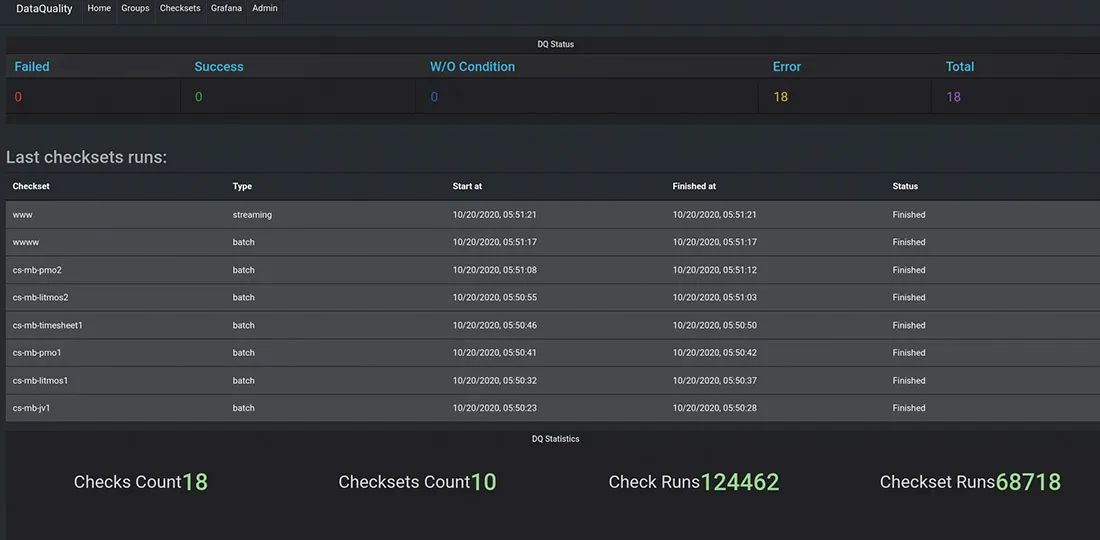
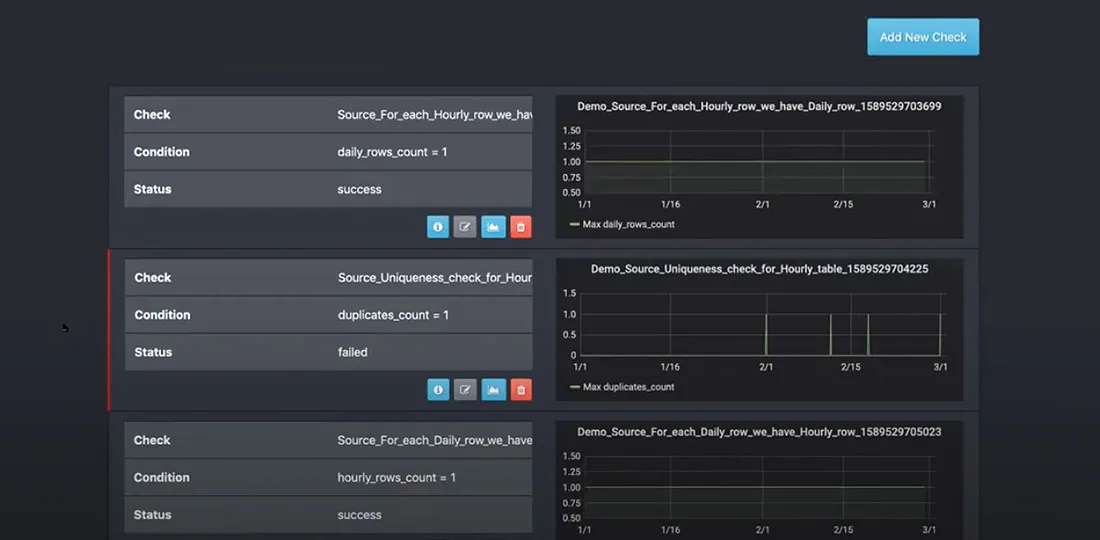
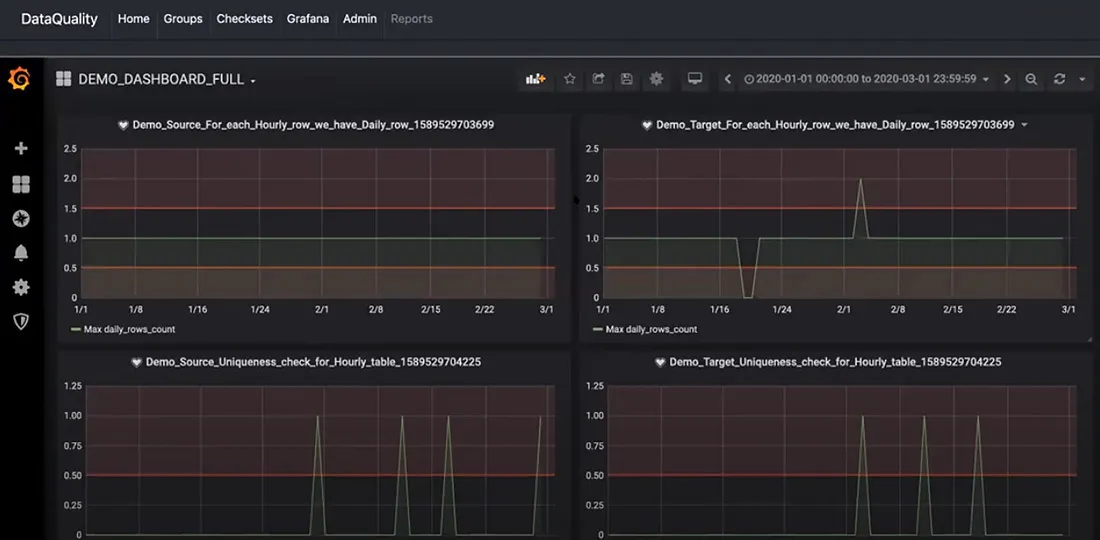
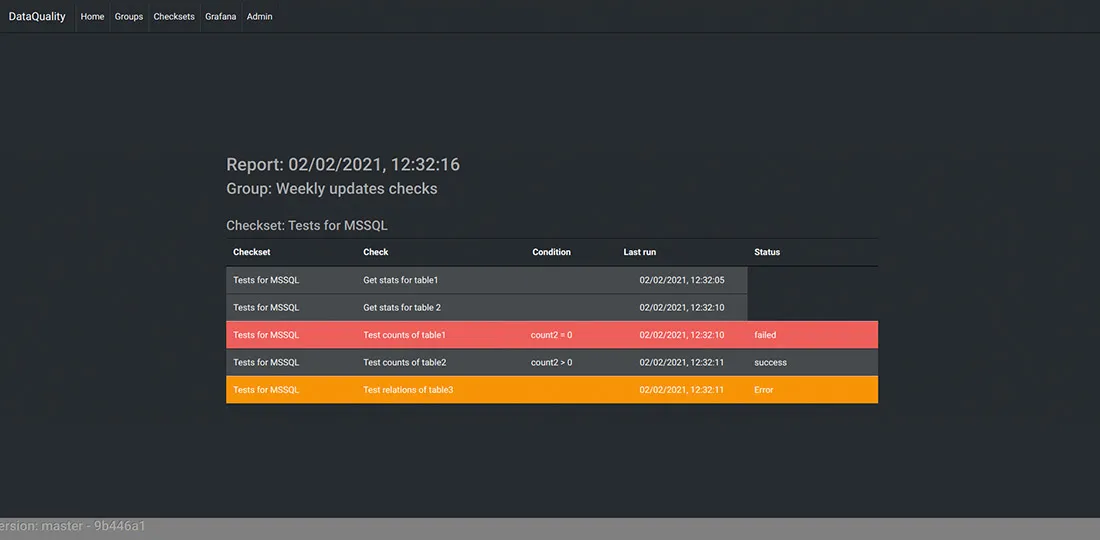
Data quality industries
We develop data quality management solutions for technology startups and Fortune-1000 enterprises across industries including media, retail, brands, gaming, manufacturing, and financial services.
Technology and media
Technology and media companies often deal with truly big data. From customer clickstream to IoT data, ensuring accuracy, completeness, and correctness of data in real time is paramount. Get access to the case study that details how we helped the #1 media company in the world to design and implement data quality best practices at a massive scale with a robust data quality management solution.
Retail and brands
Completeness and accuracy of customer data is the key success factor in retail. We have helped Fortune-1000 retailers and brands implement data quality best practices and improve data governance, leading to a 10x decrease in data quality issues. It enabled higher confidence in the decisions that machine learning models were making and increased trust in business intelligence outcomes – leading to higher conversion and customer satisfaction.
Financial services
From payment processing to banking and insurance, high quality of data is important to ensure compliance and build reliable advanced analytics capabilities. Whether it is a streaming use case or batch processing, we can help companies enhance their big data analytics and power data governance by measuring data quality in real time, fixing data quality issues in production, and improving the quality of insights.
Read more about data quality
Stop inventing excuses for poor quality data
We provide flexible engagement options to improve the data quality of your data lake, EDW, or analytics platform. We use our cloud-agnostic starter kit to decrease implementation time and cost so that you can start seeing results in just weeks.
Demo
Request a demo if you’re interested in seeing our data quality tools in action and learn more about our approach to increasing trust in data. We will connect you with our data quality experts to brainstorm your challenges and develop solutions for your implementation journey.
Proof of concept
If you can’t commit to full implementation, we recommend starting with a proof of concept. With limited investment from your side, we will integrate our tool into your data platform and you will see the results in 3-4 weeks.
Discovery
If you’re ready to improve data quality, we will take you through the entire journey. Our team of experts will identify the most critical challenges and create an implementation roadmap. We will work together to deploy our starter kit, onboard data quality, and train your team.
More data analytics solutions
Get in touch
Let's connect! How can we reach you?
Thank you!
It is very important to be in touch with you.
We will get back to you soon. Have a great day!
Something went wrong...
There are possible difficulties with connection or other issues.
Please try again after some time.